TPJ Editor choice: Welcome to the machine: how machine learning identified metabolomic changes in Brachypodium distachyon under stress
Welcome to the machine: how machine learning identified metabolomic changes in Brachypodium distachyon under stress
Mahood, E. H., Bennett, A. A., Komatsu, K., Kruse, L. H., Lau, V., Rahmati Ishka, M., Jiang, Y., Bravo, A., Louie, K., Bowen, B. P., Harrison, M. J., Provart, N. J., Vatamaniuk, O. K., & Moghe, G. D. (2023) Information theory and machine learning illuminate large-scale metabolomic responses of Brachypodium distachyon to environmental change
Plants produce an incredible array of specialized metabolites that are used to attract pollinators, deter herbivores, communicate with each other, and regulate their own growth and physiology. It has been estimated that plants as a whole produce over one million different metabolites, but investigations into these chemicals remain difficult due to an inability to identify unique compounds from metabolomic data. Since annotating mass spectrometry data involves comparing peaks to previously described standards, less than 5% of plant metabolites can be identified in untargeted metabolomic experiments.
While working as a postdoctoral researcher with Robert Last at Michigan State University, Gaurav Moghe used molecular biochemistry, mass spectrometry, and computational techniques to study the biosynthesis of specialized metabolites. This involved hand-annotating each peak obtained from the mass spectrometer, which took a substantial amount of time and labor. Once he started his own lab at Cornell University, Moghe wondered how he could improve this process using computational approaches. He and his PhD student Elizabeth Mahood found that writing Python scripts for rule-based annotations could be useful for specific experiments, but this strategy could not be applied to the broader plant metabolome, which is incredibly complex and diverse.
To overcome this hurdle, Mahood started using a new machine learning tool called CANOPUS to predict structural classes of metabolites. Mahood’s research was focused on correlating transcriptomic and metabolomic data to better annotate gene function in the model grass Brachypodium distachyon. To do this, they decided to grow B. distachyon in a wide range of conditions, including copper deficiency, heat stress, low phosphate, and with or without mycorrhizal inoculation, all with the intention of producing significant metabolomic perturbations in roots and leaves.
After obtaining mass spectrometry data from these experiments, the team then set out to understand shifts in specific metabolite classes. They initially performed spectral matching using public databases, but as expected this provided structural information for only about 5% of the queried metabolites. When they used CANOPUS, about 80% of the queried metabolites were tentatively annotated. As CANOPUS is such a new tool, they performed quality control experiments to assess the accuracy of these predictions. The accuracy of structural class predictions was not perfect and depended on the mode of ionization, the known class of compound, and specific instrumentation. They used these quality control experiments to filter their data more stringently, and after this conservative filtering the researchers were still able to assess about 40% of the peaks in their data, a huge improvement on the 5% annotation efficiency obtained using classical methods.
In order to make the metabolomic dataset accessible to a broader scientific community, the team integrated their results into an electronic Fluorescent Pictograph (eFP) browser to visualize changes in metabolite class levels across their experimental conditions (Figure). Moghe hopes this tool will enable the community to draw further conclusions from their results and facilitate the design of future comparative metabolomic and downstream validation studies.
No mass spectrometry experiment will ever give a complete view of the plant metabolome, as the extraction solvent, chromatographic method, MS parameters such as ionization mode, and downstream analysis parameters can all impact detection and quantitation of metabolites. Nevertheless, the integration of machine learning tools such as CANOPUS does lead to the annotation of a much larger percentage of the total metabolome. Moghe has begun focusing on integrating his metabolomic data with transcriptomic data, and is also working on developing tools to predict perturbed metabolic pathways directly from untargeted metabolomics data. His ultimate goal is to associate specific enzymes with metabolite classes to improve the pace of pathway discovery and facilitate metabolic engineering.
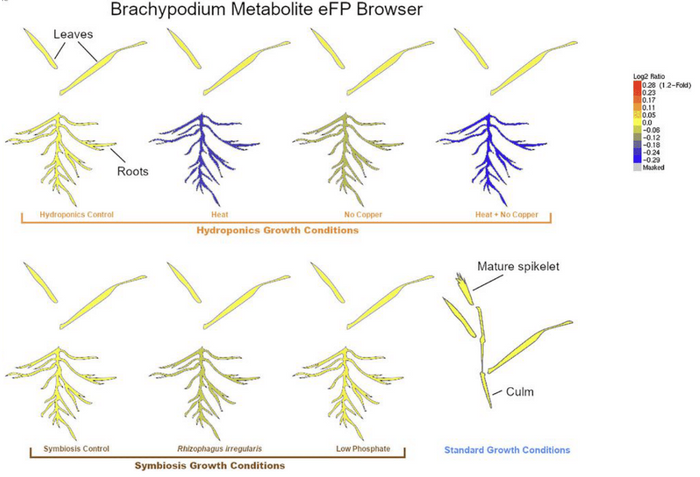
Figure: Visualizing stress-induced changes in metabolite class abundance
In the eFP browser, the log2 fold change values in metabolite class abundance (shown here for flavonoids) between a condition and its control are visualized. Note the consistent decrease in flavonoid abundance in stressed roots. Modified from Mahood et al. (2023).