TPJ Editor choice: Picture perfect: non-destructive, image-based phenotyping of bacterial wilt severity in tomato
Picture perfect: non-destructive, image-based phenotyping of bacterial wilt severity in tomato
Méline, V., Caldwell, D.L. Kim, B., Khangura, R.S. Baireddy, S., Yang, C., Sparks, E.E., Dilkes, B., Delp, E.J., & Iyer‐Pascuzzi, A.S. Image‐based assessment of plant disease progression identifies new genetic loci for resistance to Ralstonia solanacearum in tomato.
Bacterial wilt is caused by Ralstonia solanacearum, which multiplies in the xylem of roots and then grows into the shoot vasculature. The growing bacteria effectively plugs up the xylem, preventing the flow of water and causing the plant to wilt. Disease severity is typically assessed visually, but this is inherently subjective and can vary depending on who is making the assessment. Could image-based phenotyping be better?
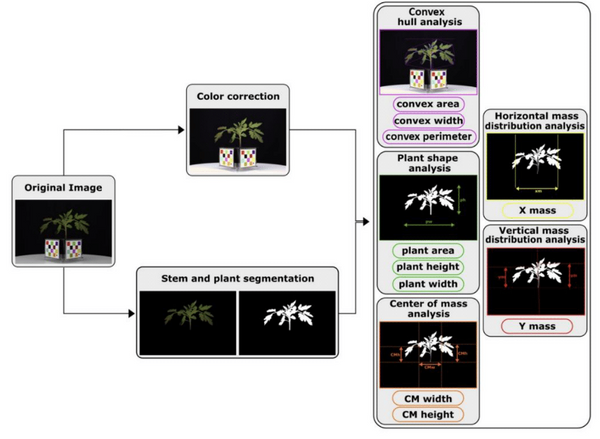
Figure: Diagram of the semi-automated analysis pipeline and 10 image descriptors.
After the original image is captured, it is color corrected and segmented to identify the stem and plant. From the color corrected image, convex area, width, and perimeter are quantified. From the segmented image, the plant area, height, width, X mass, Y mass, center of mass (CM) width and center of mass height are calculated. Modified from Méline et al. (2023).
Anjali Iyer-Pascuzzi had her first exposure to image-based phenotyping while studying changes in root architecture at Duke. But, she really wanted to focus on the intersection between plant pathology and root biology. So, when offered the opportunity to start her own lab at Purdue, she set out to find the right plant pathogen to work on. She wanted an economically important bacterial disease that infects the roots of a well-studied plant with sufficient molecular tools to allow her to make progress in a timely manner. Considering these parameters, bacterial wilt in tomato (Solanum lycopersicum) seemed like the perfect fit.
However, Iyer-Pascuzzi was frustrated by the imprecise means of phenotyping bacterial wilt disease, so she began looking for a collaborator who could extract data from images computationally. By chance, Purdue was running an initiative that encouraged agricultural faculty to work with engineering faculty. Thus, she began a collaboration with Professor Edward J. Delp, the director of Purdue’s Video and Image Processing Laboratory.
Combining their expertise, they set out to develop a pipeline for image-based, non-destructive quantification of bacterial wilt severity. To do this, they inoculated tomato plants with Ralstonia, but each of the plants was photographed the day before inoculation. The plants were then photographed again at three, four, five, and six days post-inoculation. In order to capture three-dimensional information about wilting, the plants were imaged on a turntable, with one photograph taken every 45 degrees. In total, over 40,000 images were collected. The researchers then developed a set of mathematical descriptors to assess wilting severity, settling on 10 different image-based traits to quantify different aspects of wilting (Figure).
The team then moved on to the actual goal of the study: identifying quantitative trait loci (QTLs) correlated with disease resistance. To do this, they generated a genetic map and identified 632 high-quality single nucleotide polymorphisms (SNPs). Phenotypic variation, as determined by either image-based or visual scoring, was partly explained by genotypic differences. Additionally, wilting showed a gradual increase in heritability over time, consistent with the idea that visual assessment of susceptibility was most accurate at the peak of disease severity. Using their image-based phenotyping pipeline, the team identified 27 QTLs based on the ten image-based traits. Notably, visual scoring could not identify QTLs until six days post-inoculation, while image-based QTLs were identified at three, four, and five days post-inoculation.
By identifying novel QTLs associated with bacterial wilt in tomato, the team demonstrated the applicability of non-destructive, image-based phenotyping in plant pathology research. Because the pipeline they developed is low-cost and requires minimal manual labor, Delp and Iyer-Pascuzzi are now using these methods to phenotype water-stressed plants, and hope to subsequently use the pipeline for QTL analysis of drought tolerance. They are also developing an image-based root phenotyping pipeline, since Ralstonia infects roots and changes in root architecture might be an important aspect of resistance.